Untangling the Data Mesh
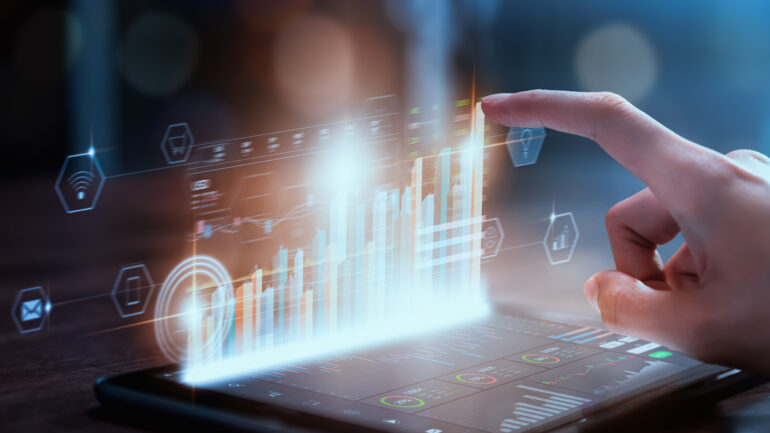
Data mesh decentralises ownership to enable a self-serve infrastructure, represents a groundbreaking shift in how organisations approach data management and directly addresses the limitations of traditional centralised models. As industries continue to generate and rely on vast amounts of data, innovative frameworks like data mesh will be critical for unlocking the full potential of data-driven decision-making.
In the 2010s, companies like Netflix and Uber faced a monumental challenge: their existing centralised data systems couldn’t scale to meet the demands of rapidly expanding user bases and increasingly complex analytics needs. Data lakes and warehouses became bottlenecks – plagued by delays, inconsistent data quality and inefficiencies caused by overburdened centralised teams.
Recognising these limitations, ZhamakDehghani, then a technologist at ThoughtWorks, introduced the concept of a ‘data mesh’. Her vision was to decentralise data ownership, empowering domain-specific teams to manage and serve their own data as products. This architecture addressed the growing need for scalability, domain autonomy and enhanced data quality, laying the groundwork for modern data innovation.
What is Data Mesh Architecture?
Data mesh is a decentralised approach to data management that prioritises domain-oriented design. Unlike centralised data lakes, where all organisational data is aggregated into a single repository, data mesh distributes data ownership across teams, each responsible for specific datasets or “data products.” This shift fosters better data quality, improved scalability and enhanced decision-making capabilities.
The Core Principles of Data Mesh
- Domain-Oriented Decentralised Data Ownership:Data is managed by the teams that generate and use it. This domain-focused ownership ensures that the data remains relevant, accurate and actionable.
- Data as a Product:Teams treat their datasets as products, emphasising user-centric design, quality assurance and accessibility. The goal is to create datasets that meet the needs of internal and external stakeholders.
- Self-Serve Data Infrastructure:A self-serve infrastructure provides domain teams with the tools and platforms needed to manage their data independently, reducing reliance on centralised IT teams.
- Federated Computational Governance:Governance is implemented at a federated level, ensuring compliance, security and consistency across decentralised datasets without stifling team autonomy.
For a data mesh to function effectively, clear roles must be defined for the individuals performing tasks within the system. Ownership is assigned to team archetypes or functions, each managing core user journeys. These roles, which can be adapted to suit the enterprise’s needs, are not always directly tied to specific employees or teams.
A data domain is typically aligned with a business unit (BU) within the organisation, such as HR, finance, or marketing with two primary domain functions: data producer teams and data consumer teams. A single data domain can serve both functions, where the producer team creates data products, and the consumer team uses these products for insights or to generate new data products.
In addition to domain-specific teams, centralised functions oversee cross-domain governance and services. These teams help manage the operational burden, ensuring compliance and facilitating inter-domain interactions essential for the mesh’s success.
Key roles within the data mesh include:
- Data Domain Producer Teams:They build and maintain data products throughout their lifecycle.
- Data Domain Consumer Teams:They discover and utilise data products for analysis or other purposes.
- Central Data Governance Team: They define and enforce policies, ensuring data quality and trustworthiness.
- Central Self-Service Data Infrastructure Team: This team provides the infrastructure and tooling for data producers and consumers.
The main pros of the architecture include:
- Scalability: Decentralised management allows organisations to handle growing data volumes more effectively.
- Improved Data Quality: Domain experts maintain their datasets, ensuring relevance and accuracy.
- Agility: Teams can respond quickly to changing business needs without waiting for centralised teams to process data.
- Enhanced Collaboration: Cross-domain collaboration is facilitated by standardised governance and infrastructure.
While benefits of a data mesh are significant, successful implementation requires overcoming several challenges. The most critical of these is cultivating a culture where teams take ownership of their data and treat datasets as products. Once this foundation is established, investing in the right tools and training is crucial for building a self-serve infrastructure – a step that many organisations hesitate to take. In implementation, achieving a balance between autonomy and compliance is essential. This balance requires careful planning and execution, ultimately determining the success or failure of the model.
Recent technological advancements have bolstered the adoption and effectiveness of data mesh architectures:
- Cloud-Native Platforms: Cloud providers such as AWS and Google Cloud now offer robust support for data mesh, enabling seamless integration of domain-specific data products across distributed environments.
- Data Observability Tools: These tools enhance monitoring and management of data quality and performance, ensuring reliability in a decentralised setup.
- AI-Powered Analytics: Artificial intelligence and machine learning tools enable domain teams to derive insights more efficiently, further emphasising the value of treating data as a product.
- API-Driven Connectivity: APIs simplify data sharing and interoperability across domains, facilitating real-time collaboration and integration.
Vertical Use Cases
Data mesh is rapidly gaining traction across verticals, demonstrating high versatility:
Financial Services: In banking and investment sectors, data mesh enables departments to manage and analyse their specific datasets, such as customer behaviour, compliance and market trends. This decentralisation enhances agility and reduces bottlenecks, improving customer experiences and regulatory compliance.
E-Commerce: Online retailers manage diverse datasets, including sales, inventory and customer preferences. Data mesh empowers domain teams to optimise operations, implement personalised marketing strategies and streamline supply chains.
Healthcare: Hospitals and research institutions use data mesh to integrate patient records, clinical trial results and administrative data. This approach improves patient outcomes by enabling tailored treatments and reducing data silos.
Manufacturing: Using data mesh, manufacturers can optimise production processes, enhance supply chain transparency and predict equipment maintenance needs through domain-specific analytics.
Government Agencies: Public sector organisations leverage data mesh for enhanced data sharing and collaboration between departments, enabling better policy-making and public services.