The Murky Waters of Machine Intelligence
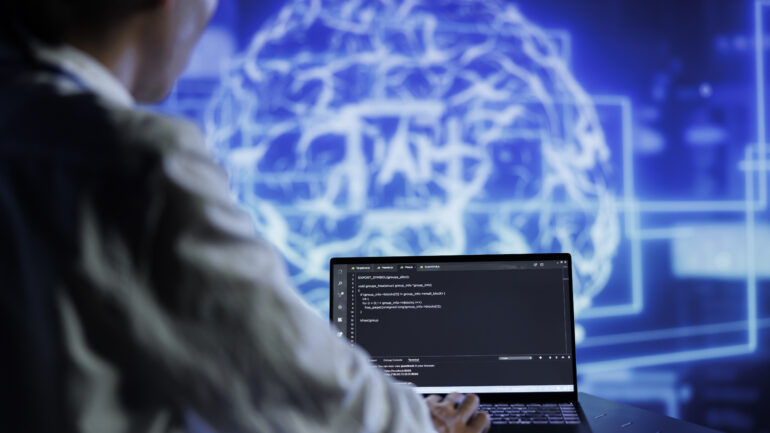
As AI continues to evolve, the black box problem remains a challenge. While explainable AI techniques offer a potential solution, they are still in the early stages of development and may not be sufficient for all applications. Organisations and regulators must work together to create frameworks that balance the need for innovation with the need for transparency and accountability.
Artificial Intelligence (AI) is rapidly transforming sectors such as healthcare, finance, and manufacturing by automating complex tasks, analysing vast datasets, and offering insights beyond human capacity. Despite these advancements, one major issue looms large: the ‘black box’ problem. This refers to the inability to fully explain how AI systems – especially deep learning models – arrive at specific decisions.
In many cases, the internal processes of these models are opaque, making it difficult for even their developers to fully understand or explain the underlying mechanics. As AI increasingly impacts our daily lives, this lack of transparency presents ethical, regulatory, and practical challenges that require urgent attention.
Decoding the Black Box
The black box problem is most often associated with machine learning models like neural networks, which mimic the structure and function of the human brain to process data. While these systems excel at pattern recognition and predictive analysis, their complexity makes it difficult to trace the decision-making path.
As the University of Michigan-Dearborn notes, these models operate like a locked vault: we can observe the inputs and the outputs, but the inner workings are hidden. This becomes problematic when AI is used in high-stakes fields such as healthcare, criminal justice, or finance, where the consequences of its decisions can directly impact human lives.
For instance, in healthcare, AI is increasingly being used for diagnostic purposes. Systems can scan medical images to detect signs of cancer or analyse patient data to recommend treatments. However, if a patient or doctor asks why a particular diagnosis or treatment plan was recommended, the answer might not be immediately available. This opacity can undermine trust, leading to hesitation in adopting AI technologies.
According to Pink Sheet, a source of regulatory insights that impact strategic business decisions, although the FDA has grown more comfortable with AI-driven medical devices, it still requires manufacturers to provide rigorous evidence of safety and efficacy, especially for systems where the decision-making process is not fully transparent. The agency’s approach highlights the tension between embracing the benefits of AI and managing the risks posed by its black-box nature.
The Innovation Dilemma
In large organisations, the black box problem becomes even more pronounced as AI-driven innovation accelerates. Companies like Google, Amazon, and IBM are pushing the boundaries of AI to streamline operations, improve customer experiences, and uncover new business opportunities. However, as enterprise innovation platform Wazoku points out, the lack of interpretability in AI models can create significant roadblocks to trust and accountability, particularly when those models are used to inform critical business decisions. For example, an AI model might recommend a certain investment strategy or flag a particular financial transaction as fraudulent, but without a clear explanation, it becomes difficult for decision-makers to act with confidence.
This issue is particularly pressing in regulated industries such as finance, where transparency and accountability are key to maintaining legal and ethical standards. AI-driven decisions that cannot be explained to auditors or regulators may result in legal challenges or fines, even if those decisions are ultimately correct. Moreover, the opacity of AI systems can exacerbate issues of bias and fairness, as it becomes difficult to determine whether the model is acting impartially or reinforcing existing prejudices.
Addressing the Black Box Problem
Overcoming the black box problem will require a multi-pronged approach. One emerging solution is the development of explainable AI (XAI) techniques, which aim to make AI systems more transparent and interpretable. XAI seeks to provide insights into how AI models make decisions without sacrificing their effectiveness. For example, instead of simply providing a recommendation, an XAI system might also explain the factors that influenced that recommendation, such as specific data points or patterns that were identified during the analysis.
However, implementing explainable AI is easier said than done. Many AI models are inherently complex, involving thousands or even millions of interconnected neurons, each contributing to the final output. Simplifying these models enough to make them understandable to humans can reduce their effectiveness, creating a trade-off between accuracy and transparency. Nonetheless, researchers and developers are working on innovative ways to strike this balance. According to Wazoku, hybrid approaches that combine human oversight with machine learning are gaining traction as a way to ensure that AI decisions are both accurate and understandable.
Global Regulatory and Ethical Considerations
The black box problem is not just a technical issue – it also has significant regulatory and ethical implications. As AI becomes more integrated into our daily lives, governments and regulatory bodies are grappling with how to ensure that these systems are safe, fair, and accountable. In the US, the FDA’s cautious approach to AI regulation reflects the broader concerns about balancing innovation with safety. While the agency acknowledges the potential benefits of AI, it also recognises the need for robust oversight to prevent unintended consequences.
Globally, different countries are taking varied approaches to AI regulation. The European Union, for example, has proposed the AI Act, which seeks to impose strict rules on high-risk AI systems, including those used in healthcare and finance. The act emphasises the importance of transparency and accountability, with provisions requiring companies to provide detailed documentation of how their AI models work and how they are tested for safety and bias. Meanwhile, countries like Japan and Singapore are adopting more flexible, model-based approaches, offering guidelines rather than hard rules.
—
Ultimately, the black box problem is a reflection of the broader challenges posed by AI; it is a powerful tool that has the potential to transform industries and improve lives, but it must be used responsibly. By prioritising transparency, safety, and ethical considerations, we can ensure that AI fulfils its promise without compromising our values or safety.