How RAG is Changing the AI Game
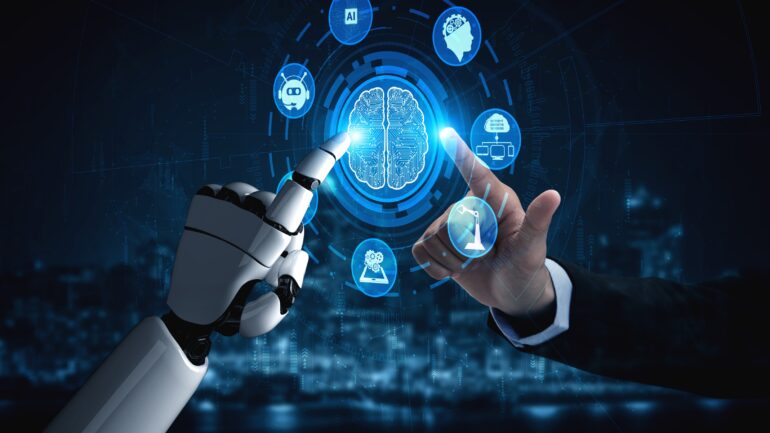
Whether you’re eight or eighty, if you’ve ever used generative AI, you’re probably amazed by its extraordinary potential to create. However, it is that very skill for fabrication that can jeopardize accuracy and sometimes, raise grave issues. Retrieval Augmented Generation, or RAG, can help with it. A crucial course correction, it tethers these potent models to reliable, verifiable data while maintaining their innovative capabilities. The outcome? A tool with increasingly boundless business potential.
When Artificial Intelligence (AI) burst onto the scene, it did so at a scale nothing short of biblical. With its expansive pronouncements, Large Language Models (LLM), current stock market favorites, show a capacity so amazing – sometimes even slightly unsettling – the dexterity with which it can carry out tasks from customer service to other creative work – chatbots write sonnets and code, generate graphics and extensive medical diagnoses all at the blink of an eye. Find me a human that can do that.
While we’ve all marvelled at the genius of creative AI, businesses now care more about how it works and the dependability of the output. Accuracy is the necessity in balance sheets, bottom lines, medical diagnoses and legal contracts.
Retrieval Augmented Generation, or RAG, is AI’s saviour. Picture it as a careful helper: one every smart but somewhat messy genius requires. Generative AI in its common form relies on the large dataset it received during training, but that dataset has limits. Though knowledge from it is considerable, it could be old, lacking a degree of nuance, prejudiced, or not suitable for a purpose. This often causes unfair or skewed results when tasks are more deterministic than creative – what people call AI ‘hallucinations.’ There are times the savant appears to make up information, delivering it with confidence similar to findings studied in detail. This becomes a huge liability.
RAG limits the volume and quantity of sources LLMs reference to give you a solution while retaining all its core skills. It does so using a retrieval mechanism to parse through only relevant documents, databases, real-time feeds etc. chosen by the user. The focus is firmly on data quality rather than the total amount in knowledge bases.
Using a predefined source list for RAG reduces the possibility of an AI delivering incorrect or old details exponentially. Results are much more reliable for technology implementation, particularly in high-risk areas like medicine or law. Additionally, RAG can also use current data sources like news, studies, or internal company data. This avoids problems from LLMs being trained on fixed datasets with data that is not the most up-to-date. For applications requiring current insight, such as market analysis or trend monitoring, this is particularly relevant. Adding new specific data to the AI’s responses without extensive retraining, which ordinarily takes a lot of time and resources, makes the model much easier to use as well.
This impact of RAG is already being felt across verticals, and rapidly. Several global companies are using it to smoothen operations and improve user experience. For example, chatbots with RAG can get current product details, FAQs along with earlier contacts to offer support that is far more precise than individual ones. This improves the applicability and dependability of AI customer service tools considerably. It is assisting doctors find applicable medical cases, research results as well as treatment instructions, plus providing more well-rounded diagnoses and suggestions. Large tech corporations like AWS, IBM, Glean, Google, Microsoft, NVIDIA, Oracle along with Pinecone are adopting RAG. Inside business settings, programs like SlackGPT have RAG incorporated to make internal knowledge management simple. Databricks uses RAG for individual information retrieval in support for customers.
Financial institutions across the globe are leveraging RAG to stay updated on latest market trends and regulatory changes, with organisations like Bloomberg using it regularly to analyse financial information. Content creation is seeing widespread changes, with RAG models helping both in research as well as fact verification, making articles and reports far better documented and accurate. Grammarly for example, uses RAG to make writing better through rewording.
But it would be prudent to remember, RAG is not a cure-all. The retrieval mechanism and the quality of data it accesses determine its power. The responses will suffer if the retrieval system does not locate the most relevant data or if the underlying data includes flaws or prejudices. Combining retrieval and generation adds another level of complexity to the procedure, potentially weighing on response times and computational demands.
—
Future development of RAG will concentrate on fixing existing issues plus augmenting current abilities. Progress, if anything, is needed in adaptive retrieval methods so they prioritize contextually significant data consistently and accurately. Multimodal RAG development, which permits information integration from a varied range of formats such as text, images and audio is yet another breakthrough that will change the game. As explainable AI garners greater traction, greater transparency into the retrieval and generation processes will be an obvious improvement.