Gap Between Expected AI Revenues & Infrastructure Investments Soars to US$600bn
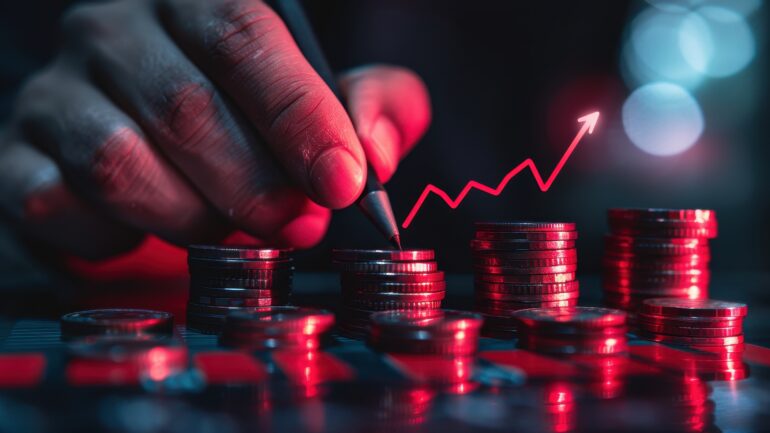
The high AI investment costs, combined with slow tangible results, has led to growing impatience among investors. And one central reason why Big Tech’s AI investments have not paid off could be the sheer cost and complexity of the technology itself.
Exactly a year ago, industry analyst and technology expert David Cahn, known for his insights on AI and market trends, posed a critical question in the article, “AI’s $200B Question“: Where is all the revenue? At that time, Cahn highlighted a significant gap between the soaring expectations surrounding AI infrastructure investments and the actual revenue growth within the AI ecosystem. This disparity was referred to as a “$125B hole that needs to be filled for each year of CapEx at today’s levels.”
Fast forward to today, and the narrative has shifted dramatically – AI’s $200bn question has now escalated to a staggering $600bn. This has impacted the valuations of Big Tech. Nvidia saw a decline of $279bn in market capitalisation, signalling that investors are becoming increasingly wary of the emerging AI technology that has driven much of this year’s stock market growth. The high cost of AI investment, combined with the slow emergence of tangible results, has led to growing impatience among investors. For instance, companies like Salesforce have faced sharp declines in their stock prices after reporting little revenue growth despite heavy spending on AI.
One of the central reasons Big Tech’s AI investments have not paid off as expected is the sheer cost and complexity of the technology itself. According to Goldman Sachs, the development and deployment of AI infrastructure – including data centres, chips, and other critical hardware – are exceptionally expensive. Jim Covello, Goldman Sachs’ Head of Global Equity Research, highlights that AI must solve very complex problems to justify its high costs. Yet, despite its potential, AI is currently not designed to address these large-scale, real-world issues effectively.
The Numbers Behind the Shift
The calculations behind this US$600-bllion figure are relatively straightforward. To understand the current landscape, one must consider Nvidia’s run-rate revenue forecast, which, when multiplied by 2x, accounts for the total cost of AI data centres. This is because GPUs constitute only half of the total cost of ownership; the other half encompasses expenses like energy, infrastructure, and backup systems. Then, to reflect the necessary gross margin for end-users – companies purchasing AI compute from Cloud providers like Azure, AWS, or GCP – this figure is multiplied by another 2x, assuming a 50% margin.
AI’s Promise vs. Performance
A significant portion of AI’s promise lies in its potential to increase productivity across industries. However, experts such as Daron Acemoglu, an MIT professor, have expressed scepticism about AI’s near-term impact on productivity and GDP growth. In a recent analysis, Acemoglu estimates that AI will only automate a limited number of tasks in the next decade, likely affecting less than 5% of all tasks across industries. As a result, he predicts only modest increases in productivity and GDP growth – 0.5% and 0.9% respectively – over the next ten years.
Changes Since September 2023
Several key factors have contributed to this dramatic increase in the revenue question:
- Supply Shortage Eases: The GPU supply shortage that plagued the market in late 2023 has largely subsided. Start-ups that once scrambled to secure GPU access are now finding it relatively easy to obtain the necessary hardware, with reasonable lead times.
- Growing GPU Stockpiles: Nvidia reported that a significant portion of its data centre revenue is now derived from large Cloud providers, with Microsoft alone accounting for approximately 22% of Nvidia’s Q4 revenue. The hyperscale capital expenditures (CapEx) are reaching historic levels, as major tech companies signal their commitment to GPU investments. However, this stockpiling could lead to a demand reset when inventories become too high.
- OpenAI’s Dominance: OpenAI continues to capture a substantial share of AI revenue, reporting an impressive growth from $1.6bn to $3.4bn in just a few months. While some startups are beginning to scale their revenues, the gap between OpenAI and other companies remains significant. Consumers are still questioning the value of AI products beyond popular applications like ChatGPT.
- Widening Revenue Gap: The previously identified $125bn hole has now expanded to $500bn. Cahn’s earlier assumptions regarding potential annual revenues from major tech companies were optimistic, but even if these projections hold, the gap is widening.
The B100 Chip and Future Demand
Nvidia’s announcement of the B100 chip, boasting 2.5x better performance at only a 25% increase in cost, is set to drive another surge in demand for Nvidia chips. This technological advancement could trigger a new supply shortage as companies rush to obtain the latest hardware.
Addressing Common Misconceptions
One of the most common rebuttals to Cahn’s previous analysis was the analogy comparing GPU CapEx to railroad construction – eventually, the “trains” (i.e., applications and services) will come. While this comparison holds some merit, it overlooks critical differences:
- Lack of Pricing Power: Unlike physical infrastructure, where monopolistic pricing can be leveraged, GPU computing is becoming increasingly commoditised. The influx of new entrants into the AI Cloud market diminishes pricing power, leading to a scenario where high fixed costs and low marginal costs can drive prices down to marginal cost.
- Investment Risks: Speculative investment frenzies often result in capital incineration, a trend observed in many technology sectors, including railroads. The lesson here is that while some companies will emerge as winners, many will falter.
- Rapid Depreciation: The pace of semiconductor innovation means that older chips, like the H100, will depreciate quickly as newer models like the B100 are introduced. This dynamic is not present in traditional infrastructure, which does not experience similar rapid technological advancements.
Looking Ahead: The Potential Remains Huge
Despite the challenges outlined, the potential for economic value creation through AI is immense. Companies that focus on delivering genuine value to end-users will be well-positioned to thrive. We are on the brink of a transformative technology wave, and companies like Nvidia are pivotal in facilitating this transition.
While speculative frenzies can be daunting, they are also part of the technology landscape. Those who maintain a level-headed approach during this period have the opportunity to build significant companies. However, we must resist the allure of the delusion that suggests instant wealth is just around the corner due to the impending arrival of AGI. The road ahead for AI will be long and fraught with challenges, but it is undoubtedly a journey worth undertaking. As we navigate this evolving landscape, a focus on sustainable growth and genuine user value will be essential for success.
Many compare AI’s current development phase to the early days of the internet. However, Covello points out that the internet, even in its infancy, provided low-cost solutions that quickly disrupted expensive incumbents. In contrast, AI technology today has yet to deliver such disruptions, especially given the monumental costs of implementation.
Big Tech’s AI investments have not yet paid off for several key reasons: the high cost and complexity of AI technology, modest productivity gains, infrastructure bottlenecks, the absence of a killer application, and mounting investor frustration. While the long-term potential of AI remains promising, it is clear that the technology’s most transformative applications may still be years away. Until then, Big Tech must navigate these challenges while continuing to invest in the future of AI, even as the path to profitability remains uncertain.