Foundation Models: Revolutionising Machine Learning and AI Applications
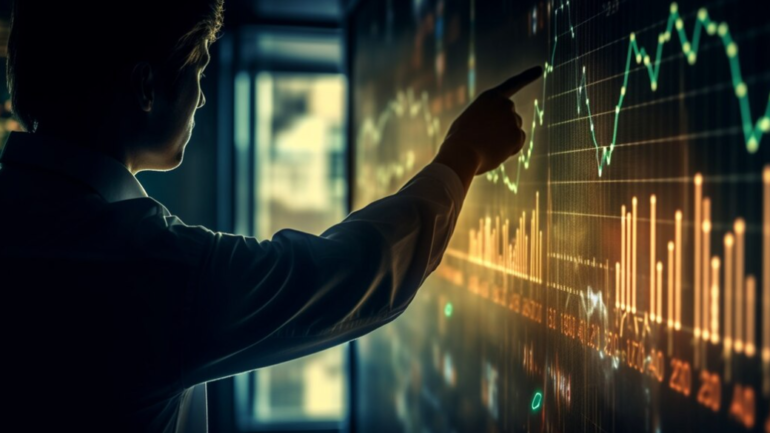
Foundation models are a cornerstone of the AI ecosystem. While challenges persist, the continued advancement and refinement of foundation models promise to unlock new frontiers in AI innovation, shaping the trajectory of technological progress in the years to come.
Foundation models (FMs) represent a groundbreaking advancement in the field of machine learning (ML), offering unprecedented capabilities and versatility. These large-scale deep learning neural networks have fundamentally transformed the approach to developing artificial intelligence (AI) applications.
Rather than starting from scratch, data scientists leverage FMs as a foundational framework, enabling the rapid and cost-effective development of diverse ML models. Coined by researchers, the term “foundation model” encapsulates ML models trained on extensive and unlabelled datasets, capable of executing a wide array of general tasks such as language understanding, text and image generation, and natural language conversations.
Evolution and Uniqueness of Foundation Models
One of the defining characteristics of foundation models is their remarkable adaptability. Unlike traditional ML models designed for specific tasks, FMs exhibit the capacity to perform a myriad of disparate tasks with remarkable accuracy, guided by input prompts. Their size and general-purpose nature set them apart from their predecessors, marking a significant departure in the ML paradigm. Data scientists can utilise FMs as base models for developing specialised downstream applications, marking the culmination of over a decade of advancement and sophistication.
For instance, the evolution of foundation models is exemplified by the progression from BERT, one of the pioneering bidirectional models introduced in 2018, to the colossal GPT-4 unveiled by OpenAI in 2023. The exponential growth in parameters and training datasets underscores the rapid pace of development in this domain. Today’s FMs, such as Claude 2, Llama 2, and Stable Diffusion, exhibit remarkable versatility, facilitating tasks ranging from content generation to image processing and dialog systems.
Foundation models have the potential to revolutionise the ML lifecycle, offering a transformative approach to developing AI applications. While the initial investment in developing FMs may be substantial, the long-term benefits are evident. Leveraging pre-trained FMs expedites the development process, enabling data scientists to deploy new ML applications swiftly and cost-effectively. Automation of tasks requiring reasoning capabilities, such as customer support, language translation, and content generation, is one of the compelling applications of foundation models across various domains, including healthcare, autonomous vehicles, and robotics.
Mechanisms and Capabilities of Foundation Models
Foundation models harness the power of generative artificial intelligence, utilising complex neural network architectures like generative adversarial networks (GANs), transformers, and variational encoders. Despite variations in network types, the fundamental principles guiding their operation remain consistent. FMs leverage learned patterns and relationships to predict outcomes based on input prompts, spanning diverse domains from text and image generation to code comprehension and human-centred engagement.
Notably, foundation models employ self-supervised learning, eliminating the need for labelled training datasets. This distinguishes them from conventional ML models, enriching their adaptability and applicability across a spectrum of tasks and domains. Their ability to learn continuously from input prompts during inference enhances their utility, enabling the development of comprehensive outputs through carefully curated prompts.
The spectrum of applications enabled by foundation models is vast and encompasses a wide range of tasks, including language processing, visual comprehension, code generation, and human-centred engagement. These models excel in natural language understanding, generating coherent responses to queries and prompts, facilitating tasks such as translation, content creation, and code evaluation.
Prominent examples of foundation models, including BERT, GPT series, Amazon Titan, AI21 Jurassic, Claude, Cohere, Stable Diffusion, BLOOM, and Hugging Face, underscore the diversity and sophistication within this domain. From text generation to image creation and code synthesis, these models epitomise the transformative potential of foundation modelling in shaping the future of AI applications.
Challenges and Considerations
Despite their remarkable capabilities, foundation models are not devoid of challenges. Building FMs from scratch entails significant infrastructure requirements and resource-intensive training processes, presenting barriers to entry for many organisations. Front-end development, including integration into software stacks and pipeline engineering, poses additional complexities for practical applications.
Moreover, foundation models exhibit limitations in comprehension and may produce unreliable or inappropriate responses, highlighting the importance of careful monitoring and oversight. Concerns regarding bias, stemming from training data and societal norms encoded within the models, necessitate proactive measures to mitigate potential biases and ensure ethical AI practices.