Fabricating Facts: The AI Hallucination Problem
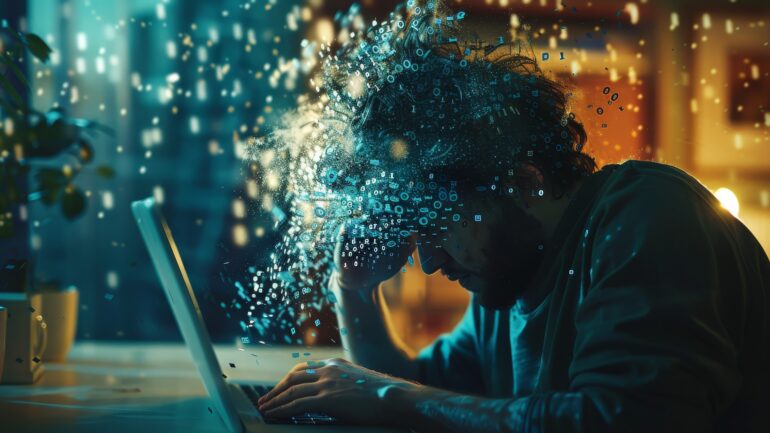
AI hallucinations pose a major challenge in the development and deployment of AI technologies. By understanding their causes and implementing effective prevention strategies, we can significantly improve the reliability and trustworthiness of AI systems, transforming them from potential sources of misinformation into dependable tools.
In a bustling hospital emergency room, a doctor consults an AI-powered medical assistant to help diagnose a patient with unusual symptoms. Unsure of the diagnosis, the doctor relies on the AI, which, trained on a vast dataset of medical records, confidently suggests a rare and complex condition, complete with treatment recommendations.
Trusting the AI’s expertise, the doctor initiates the prescribed treatment, only to watch the patient’s condition rapidly deteriorate. It soon becomes clear that the AI had produced a plausible but entirely incorrect diagnosis – a ‘hallucination’ – based on incomplete data and obscure correlations. The actual issue was far simpler, but by the time it was identified, the patient had already suffered unnecessary complications.
This scenario highlights one of the most intriguing and concerning phenomena in AI today: hallucinations. These are instances where AI models generate information that is incorrect, misleading, or entirely fabricated, yet present it as factual. Understanding why AI hallucinations occur, their potential implications, and how to prevent them is crucial for anyone working with AI technologies.
What Are AI Hallucinations?
AI hallucinations refer to instances where AI models, particularly those based on large language models (LLMs), produce output that appears plausible but is factually incorrect or entirely fabricated. These outputs can range from minor inaccuracies to entirely made-up information, such as non-existent historical events, fictional quotes, or incorrect data points. Unlike human errors, AI hallucinations occur because the model is overconfident in its incorrect output, which can be particularly dangerous in critical applications.
These hallucinations primarily result from the way AI models, especially LLMs, are trained and operate. These models are trained on vast amounts of text data, learning patterns, structures, and correlations between words. However, they do not possess a true understanding of the information they process. Instead, they predict the next word in a sequence based on probability.
AI hallucinations can manifest in various ways. Here are some common examples:
- Incorrect Predictions: An AI model might forecast an event that is unlikely to occur. For instance, a weather prediction AI could wrongly forecast rain for tomorrow despite no rain being expected.
- False Positives: An AI system may mistakenly flag something as a threat when it isn’t. For example, a fraud detection AI might incorrectly label a legitimate transaction as fraudulent.
- False Negatives: An AI model might overlook a real threat. For instance, a cancer detection AI could fail to identify a malignant tumor, missing a crucial diagnosis.
Several factors contribute to this:
- Overgeneralization: AI models can sometimes overgeneralize from the data they were trained on, leading them to make incorrect assumptions. For instance, if a model has seen many examples of a certain type of event, it might assume all similar events have the same characteristics, leading to fabricated details.
- Data Gaps: If the training data lacks information about a specific topic, the AI may “fill in the gaps” with fabricated or inaccurate information, resulting in hallucinations.
- Ambiguity in Input: When faced with ambiguous or incomplete input, AI models may generate output based on their training data’s patterns rather than factual correctness, leading to hallucinations.
- Reinforcement Learning Biases: AI models that are fine-tuned using reinforcement learning can develop biases based on the feedback they receive. If the feedback is flawed or incomplete, it can reinforce incorrect patterns, increasing the likelihood of hallucinations.
Implications of Hallucinating AI
The implications of AI hallucinations can be far-reaching, particularly as AI systems are increasingly integrated into critical domains such as healthcare, finance, and legal services. Some key implications include:
- Misinformation Spread: AI-generated hallucinations can contribute to the spread of misinformation, especially when they are presented as credible sources of information.
- Erosion of Trust:Continued AI hallucination leads to a loss in trust and reliability – a major reason forthe skeptical reactions to AI among general population.
- Ethical and Legal Risks: Inaccurate AI-generated content can lead to ethical dilemmas and legal challenges, especially if it results in harm or damages to individuals or organizations.
- Impact on Decision-Making: In fields like healthcare or finance, AI hallucinations can lead to incorrect decisions, potentially resulting in significant harm or financial loss.
How to Prevent AI Hallucinations
Preventing AI hallucinations is a complex challenge, but several strategies can help mitigate the risk:
- Improved Training Data: Ensuring that AI models are trained on high-quality, diverse, and accurate data is crucial. This reduces the likelihood of data gaps and biases that can lead to hallucinations.
- Context-Aware Systems:Developing AI systems that are context-aware can help them better understand the information they process, reducing the likelihood of hallucinations.
- Human-in-the-Loop (HITL) Approaches: Incorporating human oversight in AI decision-making processes can help catch and correct hallucinations before they cause harm.
- Continuous Monitoring and Feedback: Regularly monitoring AI outputs and providing corrective feedback can help fine-tune models and reduce the occurrence of hallucinations over time.
- Transparency and Explainability: Developing AI models with explainable outputs can help users understand how the AI arrived at a particular conclusion, making it easier to spot and correct hallucinations.
By adopting these strategies, we can significantly reduce the risk of AI hallucinations, transforming AI from a potential source of misinformation into a reliable and effective tool. Ensuring that AI systems are both accurate and trustworthy is essential for leveraging their full potential while mitigating risks.
Know more about the syllabus and placement record of our Top Ranked Data Science Course in Kolkata, Data Science course in Bangalore, Data Science course in Hyderabad, and Data Science course in Chennai.