Defrosting AI: The Race to Fix Artificial Intelligence’s ‘Frozen’ Brain
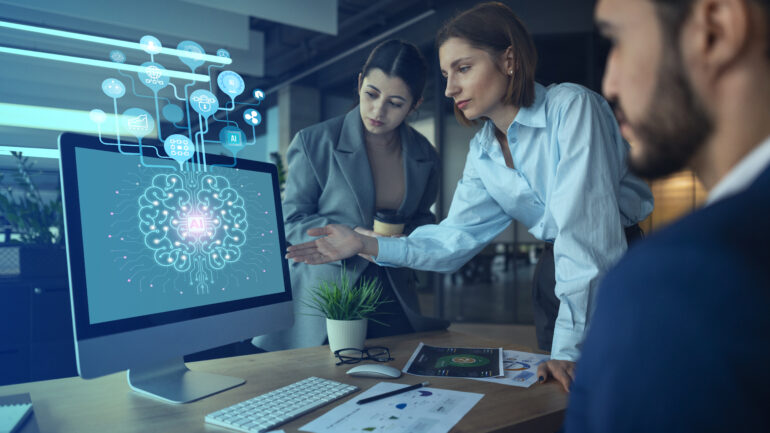
Scientists are grappling with the challenge that an AI’s learning occurs solely during training, after which its model weights remain static. Despite encountering new data post-deployment, it does not learn from them
Imagine hiring a brilliant employee who graduates top of their class, aces their training, then never learns a single new skill – or forgets how to do their job the moment they tackle something new. This is the paradox of today’s artificial intelligence: systems that can write sonnets or debug code but remain frozen in time, like a library whose books never update. Scientists are grappling with the challenge that an AI’s learning occurs solely during training, after which its model weights remain static. Despite encountering new data post-deployment, it does not learn from them.
Unlike human cognition – which learns continuously by integrating new information in real-time – AI rigidly separates training (static weights post-training) and inference phases. Eliminating this division to enable lifelong, real-time AI learning (termed continual/incremental/online learning) remains an unmet long-term research goal.
The Breakneck Rise of “Static” AI
In recent years, AI has evolved at a dizzying pace. Models like ChatGPT and Claude dazzle us with their abilities, from crafting poetry to analysing legal contracts. Yet beneath the surface lies a curious limitation: These systems learn in bursts, like students cramming for finals, rather than absorbing knowledge continuously.
“Today’s AI has two lives,” explains Dr Yujin Tang, a researcher at Tokyo-based Sakana AI. “First, it goes to ‘school’ during training – devouring terabytes of data. Then it graduates to ‘work’ during inference, applying what it learned but never growing wiser from new experiences.”
This rigid separation creates a glaring gap between artificial and human intelligence. While a child learns to ride a bike without forgetting how to subtract, AI systems face “catastrophic forgetting” – a phenomenon where new knowledge overwrites the old, like scribbling over a whiteboard until previous lessons blur beyond recognition.
The Leaky Bucket Problem
Picture trying to fill a bucket with water, only to watch it spring leaks with every new pour. That’s the challenge of catastrophic forgetting. Current AI models, built on artificial neural networks, lack the brain’s ability to strengthen some neural pathways while carving new ones.
“It’s a tug-of-war between stability and plasticity,” says Waseem Alshikh, CTO of Writer, an enterprise AI company. “Too rigid, and the model can’t adapt. Too flexible, and it forgets everything.” Traditional solutions – like periodically retraining models – are prohibitively expensive, with top systems costing hundreds of millions per update.
Nature’s Blueprint: Rivers, Not Reservoirs
Inspired by biological brains, researchers are reimagining AI as a flowing river rather than a static reservoir. Startups like Writer and Sakana are pioneering “self-evolving” models that learn organically from daily interactions.
Writer’s approach mimics human reflection. When its AI makes a mistake, it pauses to analyze the error, stores key insights in a digital “scratchpad,” and later updates its core knowledge – akin to a chef tweaking a recipe after customer feedback. “The model doesn’t just reference a manual,” Alshikh notes. “It evolves its understanding, like a musician mastering improvisation.”
Sakana’s innovation, dubbed Transformer² (or “Transformer-squared”), works differently. Imagine a team of specialists – a mathematician, a poet, a coder – embedded within an AI. When faced with a task, the system dynamically amplifies relevant experts while quieting others, allowing real-time adaptation without overwriting core skills. “It’s like having a Swiss Army knife where each tool sharpens itself as you use it,” Dr Tang explains.
Why It Matters: Beyond Smarter Chatbots
The implications stretch far beyond tech demos. Continuous learning could create AI that develops unique relationships with users – a medical assistant that deepens its understanding of a patient’s history, or a teaching tool that adapts to a student’s evolving needs.
“The models you interact with will become living entities,” predicts Anthropic CEO Dario Amodei. AI’s current “one-size-fits-all” nature makes switching between providers effortless, but personalized, learning systems could create unprecedented loyalty. Imagine a decade-old AI assistant that knows your habits better than a lifelong friend – migrating that relationship to a new system would feel like losing a part of your identity.
Despite progress, challenges remain. Rehearsing old data (like flashcards for AI) prevents forgetting but bogs down systems. Architectural tweaks risk ballooning costs. And no approach yet matches the human brain’s elegant efficiency.
Yet the race is heating up. Google DeepMind recently demonstrated a system that learns incrementally from video streams, while academic teams explore “synaptic plasticity” algorithms inspired by neuroscience. As OpenAI CEO Sam Altman observed: “The frontier isn’t just about making models bigger – it’s about making them wiser, day by day.” For now, AI remains a prodigy with amnesia – brilliant yet transient. But if researchers can bridge the gap between learning and doing, we might witness the dawn of machines that grow alongside us, turning today’s brittle tools into true partners in innovation. The key lies not in building better libraries, but in planting seeds that learn to weather life’s storms.