Transforming AI Infrastructure into Massive Digital Cities in Five years
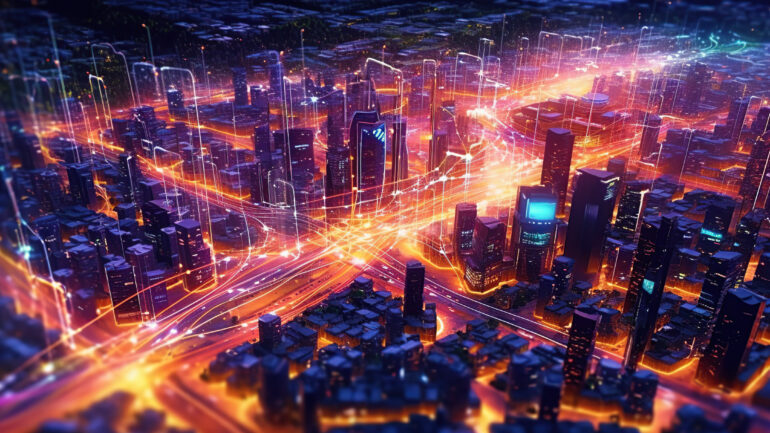
By June 2030, the leading AI supercomputer is expected to require a staggering 2 million AI chips and cost around $200 billion, akin to building a massive digital city filled with millions of tiny, powerful workers.
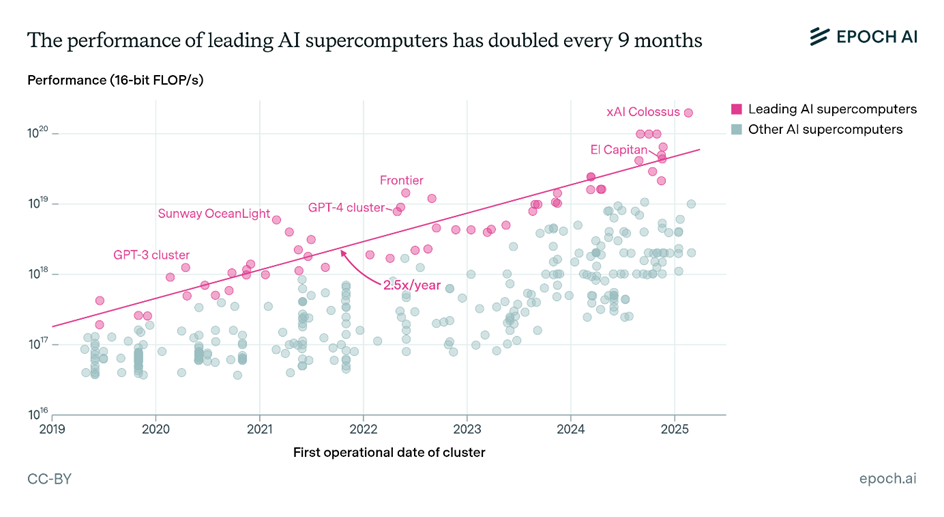
Frontier AI development relies on powerful AI supercomputers, yet analysis of these systems is limited. Researchers from Epoch AI, a research institute investigating key trends and questions that will shape the trajectory and governance of AI, created a dataset of 500 AI supercomputers from 2019 to 2025 toanalyze the key trends in performance, power needs, hardware cost, ownership, and global distribution. They found that if current trends continue then by June 2030, the leading AI supercomputer is expected to require a staggering 2 million AI chips and cost around $200 billion, akin to building a massive digital city filled with millions of tiny, powerful workers.
Meanwhile, investors are now asking when are they likely to see returns from these massive investments. According to skeptics, the AI gold rush is hitting speed bumps. After two years of breakneck spending—tens of billions poured into chips and data centers—Amazon and Microsoft are quietly scaling back. AWS has paused negotiations for overseas data center leases, while Microsoft axed two planned facilities earlier this year. Both insist it’s just “normal capacity management,” but analysts see a deeper recalibration.
However, building this AI supercomputer the real challenge lies in its power needs, which are projected to be 9 gigawatts—enough energy to run nine nuclear reactors. To put this in perspective, it’s like needing the electricity of an entire small town just to keep this supercomputer running. While the demand for chips can likely be met thanks to historical production growth and significant investments like the $500 billion Project Stargate, the sheer scale of power required could be a major roadblock.
To tackle this, companies might turn to decentralized training approaches, similar to how a large group of friends might split up tasks to get a project done faster. Instead of relying on one massive facility, they could distribute the workload across multiple AI supercomputers in different locations, ensuring that the energy demands are more manageable and that progress in AI can continue without being stifled by infrastructure limitations.
Konstantin F. Pilz, James Sanders, Robi Rahman, Lennart Heim, the troika of researchers. found that the computational performance of AI supercomputers had doubled every nine months, while hardware acquisition cost and power needs both doubled every year. The leading system in March 2025, xAI’s Colossus, used 200,000 AI chips, had a hardware cost of $7B, and required 300 MW of power, as much as 250,000 households. As AI supercomputers evolved from tools for science to industrial machines, companies rapidly expanded their share of total AI supercomputer performance, while the share of governments and academia diminished.
As AI development has attracted billions in investment, companies have rapidly scaled their AI supercomputers to conduct larger training runs. This caused leading industry systems to grow by 2.7x annually, much faster than the 1.9x annual growth of public sector systems. In addition to faster performance growth, companies also rapidly increased the total number of AI supercomputers they deployed to serve a rapidly expanding user base. Consequently, industry’s share of global AI compute in our dataset surged from 40% in 2019 to 80% in 2025, as the public sector’s share fell below 20%
Globally, the United States accounts for about 75% of total performance in our dataset, with China in second place at 15%. If the observed trends continue, the leading AI supercomputer in 2030 will achieve 2×1022 16-bit FLOP/s, use two million AI chips, have a hardware cost of $200 billion, and require 9 GW of power. The Epoch analysis provides visibility into the AI supercomputer landscape, allowing policymakers to assess key AI trends like resource needs, ownership, and national competitiveness.