Open Innovation’s Cybersecurity Reckoning
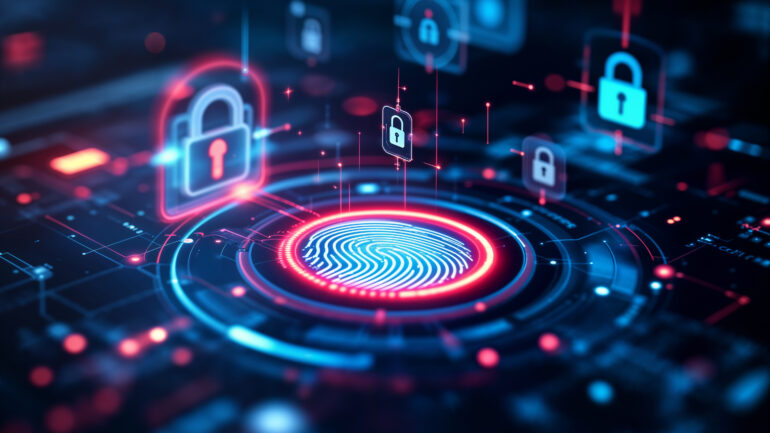
Artificial Intelligence, surging at a pace unprecedented, is today accelerating Open Innovation to heights unseen. This potent genie does, however, come with a Faustian twist: an increase in novel and intricate cyberthreats. Overlooking strong governance is no longer an option; doing so will only lead to economic instability and trust erosion.
It is nothing short of groundbreaking how AI is being incorporated into today’s open innovation (OI) engine. Much like the steam engine or the internet did before it, AI’s scalability and analytical capabilities promises to transform industries, speed up discoveries and unleash previously unheard-of efficiency. Shared data, sophisticated algorithms and a common drive for creativity that cuts across corporate borders are pushing a Cambrian boom of collaborative ecosystems. However, as with any technical advancement – while innovation will have a lasting impact, the quick adoption of AI in OI has already resulted in a new set of cyberthreats that require our immediate attention and careful management.
The phrase ‘open innovation’ – described by the Oxford Review – refers to a situation in which an organization uses a variety of external sources, including published patents, competitors, external agencies, and customer feedback in addition to its own internal knowledge, sources, and resources (such as its own employees or research and development, for instance) to drive innovation in products, services, business models, processes, etc.
Essentially, OI is about opening up processes and bringing in external resources and experience. This openness is enhanced by AI making possible the analysis of more varied datasets in-depth and collaboration on more projects with greater complexity. However, this very transparency, made much more so by AI’s use, makes it ripe for abuse. The conventional walls and moats that organizations depended on for protection become porous and rife with fresh weaknesses that bad actors are already keen to exploit.
Data security and privacy concerns are unsurprisingly among the most pressing issues. In collaborative OI environments, sharing or pooling sensitive data is frequently necessary for AI models to thrive. As experts at the California Management Review recently point out, federated learning is vulnerable to “data poisoning” — the insidious introduction of tainted data specifically aimed at ruining model performance. Federated learning is a promising technique that enables collaborative training without sharing raw data. Likewise, although synthetic data mimicking real-world datasets appears to be a safer option, its manipulation might still reveal hidden flaws. Similarly, generative AI systems present their own vulnerabilities – if their training data is compromised, it could result in infringements of intellectual property essential to innovation, for example. Even seemingly-innocuous activities such as web-scraping training data can inadvertently pull in sensitive or proprietary information.
In addition to the issue of the data itself lies the delicate issue of algorithmic integrity. AI models are far from impenetrable. The threat of backdoors, a digital Trojan horse that attackers can use during model training, can alter outputs and impair performance. Consider the ramifications for financial OI, where a compromised AI model may quietly change risk evaluations, opening the door for extensive fraud. The success of OI thus depends critically on trust in these AI-driven systems, which themselves can be quickly destroyed by hacked algorithms. Strong auditing and validation procedures, similar to the checks and balances we need in our financial institutions, are now essential to preserving algorithmic robustness and transparency.
Furthermore, it is the very nature of collaborative ecosystems in AI-powered OI that makes it susceptible to attacks. Platforms that facilitate such features need to be airtight – closing the door to malicious actors who can distribute counterfeit content or embed malware that can cause a quagmire of legal disputes and reputational damage. Compromised datasets resulting in flawed output or the theft of intellectual property are the primary concerns here. Plus, the interconnectedness of systems that fuel innovation also, on the flip side, amplifies the potential for contagion.
While potential issues have been diagnosed in abundance, a prescription for the cure is not yet foolproof. The California Management Review offers a three-pronged framework for Cyber Risk Governance (CRG):
Firstly, firms need to prioritize dealing with AI-associated threats by carefully mapping out important AI resources – datasets, algorithms and collaboration tools and their dependencies – within the OI ecosystem. “Crown jewels,” vital data warehouses, need to be the focus, as these contain crucial AI model training settings and proprietary synthetic data generators. Well-known ISO/IEC 42001 or NIST AI Risk Management frameworks, modified for threats like data poisoning and hostile attacks, offer a useful roadmap.
Second, businesses need to effectively measure AI cyber risks and go beyond the qualitative. Translating often technical hazards into operational consequences and reputational harm is necessary for communicating CRG effectively. A solid approach is provided by scenario modeling with programs such as the Factor Analysis of Information Risk for Artificial Intelligence Risks (FAIR-AIR). A significantly more powerful message for executives than merely claiming that it is a “high risk” is to explain that a corrupted AI model might result in a multi-million dollar regulatory sanction.
The third prong and probably the most important – companies need to concentrate on creating and maintaining strong governance frameworks right from the start. This means allocating explicit responsibilities for AI-related cyber risks across all organizational levels. It is now essential – rather than optional – to enable key C-suite positions, including as CISOs, CIOs, CDAOs, CPOs, and OI business executives, to incorporate cybersecurity into AI innovation strategies. Specialized committees to specify AI risk tolerance and matching cybersecurity protocols with innovation objectives is key.
–
AI risk governance must be adaptable; rules must take audit findings into account and evolve to keep up with emerging threats and technological advancements. Monitoring key performance indicators (KPIs) that focus on model dependability, compliance, and risk mitigation-return on investment (ROI) is necessary to assess efficacy.
Cybersecurity measures suited to ethical AI practices and encouraging collaborative security inside innovation ecosystems through multi-stakeholder governance frameworks, are essential to boost collective resilience in accordance with the true spirit of OI. Read “Cyber Risk Governance (CRG) in the Age of AI-Driven Open Innovation” by the California Management Review (April 1, 2025) here.