The AI Gold Rush’s Reckoning: What If Efficiency Topples Big Tech’s $600 Billion Bet?
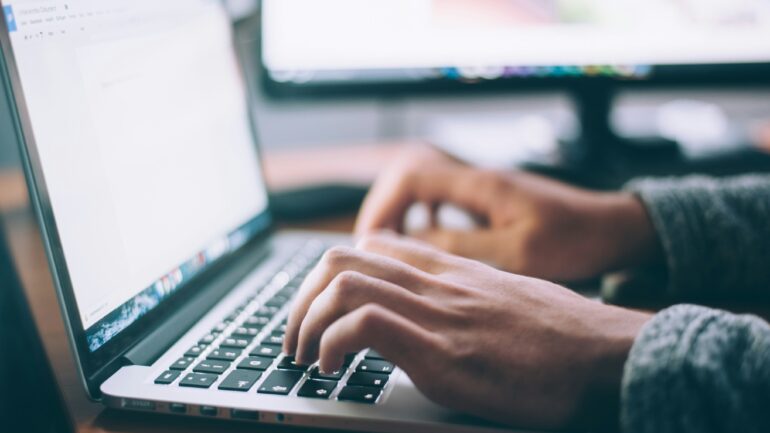
The red flag raised mid last year, has now become a harsh reality as DeepSeek challenges the paradigm of the muscularity of mega investments vs. smarter algorithms. Will 2025 be remembered as the year AI’s future was forged not by those who built the biggest machines, but by those who taught them to think leaner?
The troubling question was first raised by Sequoia Capital in an article back in June 2024. It was about the stark gap between massive infrastructure investments in AI by the US Big Tech and the alarming gap in actual revenue generation from this capex. The assumption that tech behemoths like Google, Microsoft, Apple, and Meta can generate $10 billion annually from AI-related revenue appeared increasingly optimistic even then. With projections now indicating a $500 billion gap, questions about the feasibility of these targets are coming in thick and fast. The red flag raised mid last year, has now become a harsh reality as DeepSeek challenges the paradigm of the muscularity of mega investments vs. smarter algorithms.
Today this has become the scenario. The neon-lit data centres of America’s tech giants hum with an almost religious fervour in 2025, their aisles of GPUs consuming enough electricity to power mid-sized nations. Microsoft, Meta, and Alphabet are collectively pouring over $280 billion this year into AI infrastructure – a sum larger than Hungary’s GDP–betting that artificial intelligence will justify history’s most expensive science experiment. Yet 6,000 miles east, a Chinese startup named DeepSeek is quietly demonstrating that the future of AI might not belong to those with the deepest pockets, but to those with the smartest algorithms.
This collision of philosophies–Silicon Valley’s “bigger is better” ethos versus Shenzhen’s “smarter is sustainable” approach–has exposed a $600 billion question: What happens if brute-force spending becomes obsolete before it pays off?
The Capex Abyss
Microsoft’s $80 billion AI infrastructure budget for 2025 could purchase 13 nuclear aircraft carriers. Meta’s AI R&D costs are rising 22% annually despite generating less than 8% of revenue. Analysts now warn of a $500 billion annual chasm between what US tech titans spend on AI infrastructure and what the technology actually earns–a gap widening faster than the Mojave Desert during drought season!
The math grows more ominous by the quarter. For every dollar Microsoft makes from AI services, it spends $6 preparing infrastructure to make more. Nvidia’s record GPU stockpiles gather dust in Nevada warehouses as Cloud providers slash AI service prices by 20%, trapped in a race to the bottom. Even Wall Street’s patience wears thin; when DeepSeek debuted its ultra-efficient model in March, Nvidia lost $600 billion in market value in a single day, equivalent to erase Starbucks, Ford, and Pfizer from existence overnight.
The Efficiency Paradox
DeepSeek’s breakthrough reads like a Silicon Valley parody: A $5.8 million system (0.3% of OpenAI’s compute budget) matching GPT-4’s capabilities while using less energy than a suburban shopping mall. Their secret? Treating AI development like Olympic weightlifting–maximising output through precision engineering rather than sheer mass.
By combining open-source collaboration, hybrid chipset architectures, and radical model compression techniques, the Chinese firm achieved what US giants dismissed as fantasy: 92% hardware utilisation rates versus the industry’s 65% average. While American data centres guzzle 500+ megawatts–enough to dim San Francisco’s streetlights–DeepSeek’s system sips power at levels that wouldn’t strain a provincial university’s electrical grid.
“This isn’t just about cost,” notes Stanford AI researcher DrLila Rao. “It’s about exposing the fallacy that progress requires exponentially more resources. DeepSeek proves we’ve been solving AI equations with the wrong variables.”
The Sustainability Cliff
The environmental stakes transform this from boardroom drama to planetary challenge. US AI infrastructure now consumes 4.3% of national electricity, triple 2022 levels, with projections hitting 12% by 2027. Each ChatGPT query already emits 20 times more CO₂ than a Google search. At current growth rates, the AI sector could single-handedly add 1.5°C to global warming by 2035.
DeepSeek’s model offers a 40% reduction in this trajectory, but adoption faces cultural resistance. American tech culture remains wedded to Moore’s Law orthodoxy–the belief that throwing more transistors (and dollars) at problems guarantees solutions. Meanwhile, China’s constraints (US chip sanctions, energy scarcity) forced innovation through necessity.
Three Roads From Here
Industry analysts see diverging paths:
- The Efficiency Domino Effect (60% Likelihood): If DeepSeek’s architecture becomes the 2026 standard, demand for Nvidia GPUs could collapse 70%, triggering $1 trillion in Big Tech infrastructure write-downs. Cloud providers pivot to leasing AI efficiency as a service.
- The Hybrid Compromise (30%): Microsoft and Google license DeepSeek-like systems for routine tasks while reserving heavyweight models for premium services–an AI caste system. Profit margins contract as customers refuse to pay luxury prices for marginally better email drafting.
- The Capex Implosion (10%): The $500 billion annual investment-revenue gap triggers a 2026 valuation crisis. AI stocks mirror 2022 crypto collapses, with Meta and Alphabet losing $300 billion combined in a quarter. Venture funding freezes for a generation.
The Uncomfortable Mirror
This crisis reflects a deeper Silicon Valley identity struggle. For decades, tech’s winners were those who scaled fastest–Facebook in social networks, Amazon in Cloud computing. AI was supposed to follow the same playbook. But as Microsoft spends $80 billion chasing AI revenue that may never cover its capital costs, the industry faces an existential query: What if the next technological revolution rewards conservation over consumption?
The clock ticks louder each quarter. US tech giants have committed $1 trillion in AI infrastructure through 2027, assuming annual revenue growth rates triple current levels. Yet every percentage point Drop in GPU demand shaves $8 billion off Nvidia’s valuation. Every watt saved by efficient algorithms weakens the rationale for building 500-megawatt data centres. As the sun sets over Microsoft’s Arizona data complex, its cooling towers steaming like industrial-era relics, one wonders whether 2025 will be remembered as the year AI’s future was forged not by those who built the biggest machines, but by those who taught them to think leaner. The coming months may determine whether “move fast and break things” gives way to a new mantra: “Work smart and sustain.”