Zero-Shot and Few-Shot Learning Redefine the Limits of AI
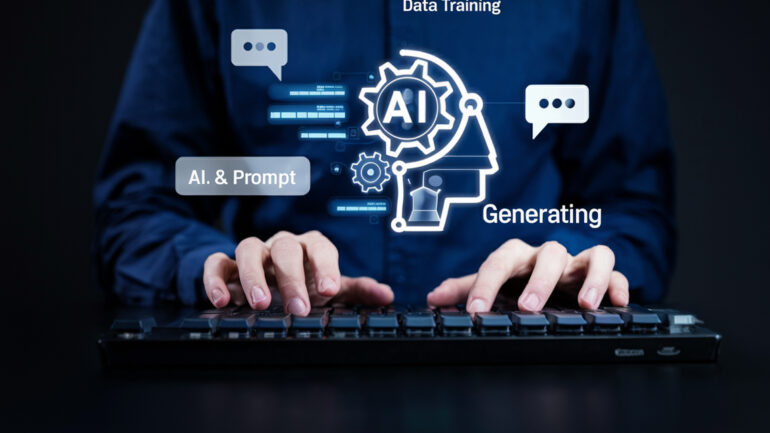
Zero-shot and few-shot learning are not just technological milestones; they represent a philosophical shift in how we approach AI: away from data-hungry systems to more efficient, adaptable models. As industries and researchers explore these paradigms, the goal remains to build AI systems that are powerful yet ethical, efficient, and inclusive.
Artificial intelligence (AI) stands at a crossroads. As the demand for smarter, more adaptive systems grows, traditional methods of training AI on massive, labelled datasets face practical and ethical challenges. Enter zero-shot learning (ZSL) and few-shot learning (FSL), two techniques poised to revolutionise how machines learn, adapt, and generalise. These approaches aim to mimic human-like learning by achieving high performance with little to no specific training data. Their implications stretch across industries, promising breakthroughs while raising complex questions about scalability and trust.
The explosion of generative AI models like OpenAI’s ChatGPT and Google’s Bard (Gemini) has underscored the importance of generalisation. In 2025, the buzz surrounding ZSL and FSL is not just academic – it’s deeply practical. These methods enable AI to tackle novel problems without extensive retraining, making them invaluable for industries grappling with data scarcity, like healthcare, legal analysis, and emerging markets.
However, the urgency is driven by more than utility. The EU’s AI Act and similar regulations worldwide have heightened scrutiny over how AI models are trained. Ethical AI systems, particularly those that reduce biases and dependency on vast labelled datasets, are more relevant than ever.
From Data Hunger to Data Efficiency
Traditional AI models relied heavily on large, labelled datasets. Supervised learning, the most widely used paradigm, required painstaking annotation, often outsourced at scale. For instance, labelling millions of images or classifying text was time-intensive, expensive, and prone to human error.
Few-shot learning emerged as a solution, inspired by humans’ ability to learn from limited information. Early models like Siamese Networks demonstrated that even a handful of examples could suffice for robust predictions. The idea was simple: focus on relationships and similarities rather than sheer volume.
Zero-shot learning, on the other hand, took a more audacious leap. Instead of relying on examples, ZSL models generalised from semantic information. By leveraging embeddings – mathematical representations of data – ZSL aligned unseen categories with those it already knew. For instance, if a model understood “horse” and “zebra,” it could infer the concept of “donkey” without explicit training.
While both methods aim to make learning more efficient, they differ in their approach and scope:
- Few-Shot Learning (FSL): Trains on a limited number of labelled examples for a specific task. Ideal for applications like rare disease detection or identifying uncommon defects in manufacturing.
- Zero-Shot Learning (ZSL): Does not train on task-specific data but uses prior knowledge and semantic understanding. Often employed in NLP for tasks like sentiment analysis or text classification in novel domains.
The Mechanics of Learning with Less
At their core, ZSL and FSL benefit from advances in transfer learning and embeddings. Transfer learning involves reusing knowledge from one task to solve another. For instance, a model trained to recognise cats and dogs can be repurposed to identify lions with minimal additional data. Embeddings create a shared representation space, allowing models to understand relationships between known and unknown categories.
This synergy is best exemplified in Transformer-based architectures like BERT and GPT, where self-supervised learning enables models to predict outputs with minimal human-labelled data. These architectures form the backbone of ZSL and FSL, making them highly versatile.
Some of the major applications include:
- Natural Language Processing (NLP):Large language models (LLMs) like OpenAI’s GPT-3 epitomise few-shot learning. In tasks like text summarisation or translation, these models adapt seamlessly when given just a few contextual examples. Zero-shot learning, by contrast, shines in question-answering systems where the model derives answers without specific task training, relying on its broader linguistic understanding.
- Healthcare Diagnostics: Consider diagnosing rare diseases. Collecting a comprehensive dataset for such conditions is nearly impossible. Few-shot models trained on limited patient data can detect anomalies with high accuracy, while zero-shot models extend these insights to previously un-encountered diseases using shared medical features.
- E-commerce Personalisation:Retail platforms leverage FSL to predict customer preferences using minimal interaction data. Zero-shot learning expands this capability by understanding new product categories or customer behaviours without retraining, improving recommendations in real-time.
- Autonomous Vehicles:FSL aids self-driving cars in identifying rare or dangerous scenarios with sparse labelled examples. Zero-shot learning augments this by recognising entirely new objects – like unexpected road hazards – by correlating them with known patterns.
The future of zero-shot learning (ZSL) and few-shot learning (FSL) is intertwined with the quest for greater generalisation and adaptability in artificial intelligence. A key direction is the development of cross-domain learning capabilities, where AI models can fluidly switch between entirely different tasks. Imagine an AI that can seamlessly translate text from one language to another and then analyse visual content within the same operational context. This level of versatility could redefine the scope of AI applications, enabling systems to transcend the constraints of domain-specific training.
Another transformative avenue lies in the integration of synthetic data. By generating artificial datasets that mirror real-world scenarios, researchers can address the challenges of data scarcity and bias. Synthetic data allows for the creation of diverse training environments, enabling ZSL and FSL models to perform with greater accuracy and reliability in dynamic and unpredictable conditions.
Quantum machine learning presents yet another exciting frontier. As quantum computing technologies mature, they hold the potential to supercharge ZSL and FSL through enhanced optimisation of embeddings and transfer learning algorithms. By leveraging quantum mechanics, these models could achieve unprecedented speed and precision, opening up possibilities for handling complex data structures and tasks that are currently beyond reach.
Equally important is the role of ZSL and FSL in promoting AI for social good. These methods have the potential to democratise access to advanced AI, particularly in resource-constrained settings. From disaster management systems that analyse limited real-time data to educational tools tailored for underserved communities, these learning paradigms can bridge critical gaps. They offer a pathway to create AI systems that are not only innovative but also inclusive, addressing pressing global challenges with minimal resource requirements.
Together, these advancements mark a profound shift in how AI learns and operates, paving the way toward more adaptable, efficient, and equitable systems. Yet, despite their promise, ZSL and FSL are not without hurdles:
- Bias Propagation:Generalisation can amplify biases present in the training data. For example, a zero-shot model might incorrectly classify novel inputs based on stereotypes embedded in its semantic understanding.
- Reliability: Models may falter in high-stakes applications like medicine or autonomous driving, where errors have serious consequences. Ensuring confidence in predictions remains a critical challenge.
- Resource Intensity:While these methods reduce the need for labelled data, the initial training of foundational models is computationally expensive, often requiring significant resources.
- Interpretability: Both ZSL and FSL operate on complex relationships that are difficult to interpret, raising questions about transparency and trust.
Zero-shot and few-shot learning represent a paradigm shift in artificial intelligence, blending innovation with necessity. They have demonstrated the potential to revolutionise industries, bridge resource gaps, and address global challenges with precision and adaptability. Yet, their true power lies in their capacity to inspire a broader vision for AI – one that transcends data constraints and pushes toward a more generalised intelligence.