Breaking Down Hurdles to Enterprise GenAI Adoption
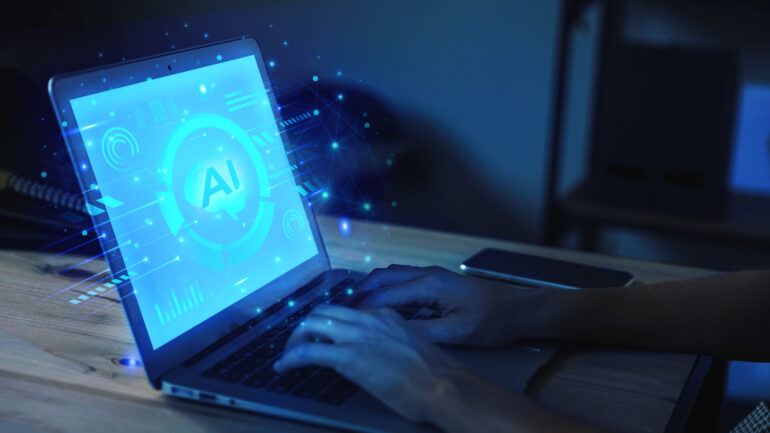
The ability to adapt to technological change depends not just on innovation but also on the institutions that support it. For GenAI, the potential is limitless, but only if enterprises are willing to dismantle the hurdles that hold them back
Generative AI (GenAI) represents one of the most transformative technologies of our era. Yet, for enterprises, the journey from innovation to integration has proven unexpectedly challenging. Recent Morgan Stanley research sheds light on the intricacies of this adoption process, identifying key hurdles such as data security, organisational buy-in, and regulatory concerns.
Data Security – The Invisible Barrier
Data security has rapidly become the primary concern in the adoption of GenAI, particularly for organisations that manage sensitive customer data or intellectual property. According to Morgan Stanley’s proprietary AlphaWise survey of 400 companies, an overwhelming 60% of respondents listed data security as their foremost concern. This statistic underlines a widespread anxiety – one that transcends industry boundaries and encompasses sectors ranging from healthcare to finance. For these industries, the stakes are even higher. The potential for data breaches, misuse or even inadvertent exposure of confidential information could result in catastrophic consequences, both in terms of financial losses and reputational damage.
The trepidation surrounding data security is not misplaced. While the scale of data that needs to be processed and stored grows exponentially, it is not merely that which creates vulnerabilities, it is the inherent complexity in managing and protecting such vast amounts of information that is most susceptible. Traditional methods of securing data are often ill-equipped to handle the sophisticated and dynamic nature of GenAI systems, necessitating the development of advanced security protocols to safeguard against emerging threats.
Walmart’s recent foray into GenAI for catalogue updates serves as a notable example of the trade-off between innovation and security. The retailer now processes over 850 million pieces of data nearly 100 times faster than before, thanks to GenAI’s capabilities. However, the company’s success hinges on more than just cutting-edge technology – it is the robust data security framework that underpins the entire operation. The need for encryption, differential privacy, and stringent access controls is non-negotiable. These measures are essential not only for ensuring the integrity of sensitive data but also for adhering to regulatory standards and maintaining consumer trust.
For enterprises integrating GenAI into the crux of their operations, the imperative to address data security is clear. Those that proactively invest in advanced encryption methods, use differential privacy techniques and enforce strict access controls will not only mitigate risks but will also gain a competitive advantage.
Enterprise Culture – The Achilles’ Heel
Even with clear value propositions, GenAI projects often struggle to secure organisational buy-in. Nearly half of surveyed companies reported difficulties in formulating a coherent AI strategy or obtaining management approval. For many traditional organisations, AI adoption threatens to disrupt long-standing workflows and hierarchies.
A contrasting example comes from Salesforce. Its “Agentforce” platform democratises automation by enabling users to set up AI agents without coding expertise. This solution not only simplifies automation but also garners wider acceptance by aligning with employees’ existing skill sets. Prioritising human-centred design and offering hands-on training, Salesforce overcame resistance and positioned itself as a GenAI leader.
Yet, haste to implement GenAI without due diligence can backfire, as evidenced by 25% of companies citing reputational risks as a major deterrent. Legal issues, including copyright and compliance, further exacerbate the challenges. This is particularly critical in industries with stringent regulations like pharmaceuticals or consumer goods.
L’Oréal’s “Skin Genius” exemplifies a cautious yet effective approach. Using AI to recommend skincare products, L’Oréal achieved a remarkable increase in conversion rates – from 10% to over 70% at its counters. The tool adheres to data privacy regulations and ensures ethical AI practices, thereby mitigating reputational risks.
Despite challenges, there are enough success stories to offer a roadmap for others.
- Meta’s Advantage: Meta leveraged GenAI to enhance advertising personalisation, resulting in a 22% higher return on ad spend. Its structured experimentation with generative models allowed the company to scale while minimising risks.
- Axon’s Draft One: Law enforcement agencies using Axon’s GenAI tool reported a 30% reduction in time spent on report writing. Incorporating clear guardrails, Axon ensured that its AI was both effective and trustworthy.
- Synopsys.ai: Automating repetitive semiconductor design tasks, Synopsys achieved a 20% rise in contract sizes, underscoring how key aligning GenAI capabilities with specific business needs is.
To transition GenAI successfully from proof-of-concept to production, enterprises must adopt a multi-faceted strategy:
- Strengthen Data Governance: Organisations must invest in secure data pipelines, implement robust compliance frameworks, and foster transparency.
- Foster Cultural Change: Executives need to articulate clear AI strategies and provide incentives for cross-functional collaboration.
- Engage in Responsible Innovation: Enterprises should prioritise ethical considerations and involve diverse stakeholders in the development process.
GenAI is not a one-size-fits-all option. Its true promise will be realised by those enterprises that understand transformation is not just about adopting new tools, but about evolving their very way of working. Ethical, responsible innovation coupled with an environment that supports both technological and cultural shifts is how companies can break through the barriers to adoption and position themselves at the forefront of the AI-driven future.