Illuminating the ‘Black Box’ with Explainable AI (XAI)
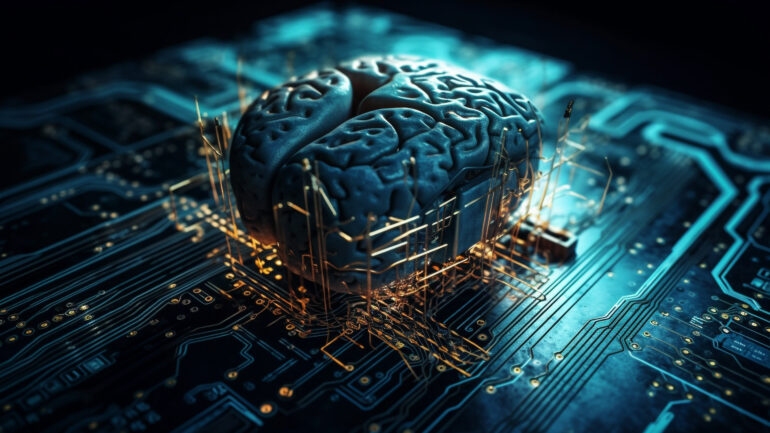
Explainable AI is not merely a technical enhancement but a fundamental requirement for the responsible and effective deployment of AI in today’s complex and regulated business environment. Its adoption will distinguish forward-thinking organisations that are prepared to navigate the challenges and opportunities presented by the AI revolution.
Artificial intelligence has reached a pivotal moment, where its growing influence demands more than just performance – it calls for transparency and trust. Enter Explainable AI (XAI), a paradigm designed to demystify AI systems by elucidating internal mechanics, underscored by recent regulatory developments, notably the European Union’s General Data Protection Regulation (GDPR), which mandates transparency in automated decision-making processes.
The Mayo Clinic, for example, implemented an AI system for predicting patient outcomes and recommending treatments. To ensure trust and adoption among medical professionals, the system utilised XAI techniques to provide clear explanations for its predictions. This transparency enabled doctors to understand and trust the AI’s recommendations, leading to improved patient care and outcomes.
The necessity for XAI is multifaceted, encompassing trust, accountability, compliance, and performance enhancement:
- Building Trust: Transparency in AI decision-making processes fosters trust among users, stakeholders, and regulators. When users comprehend how an AI system arrives at its conclusions, they are more inclined to trust and adopt the technology.
- Ensuring Accountability: XAI enables organisations to hold AI systems accountable for their decisions, a critical aspect in high-stakes domains such as healthcare, finance, and criminal justice.
- Regulatory Compliance: With regulations like the GDPR requiring transparency in automated decision-making, XAI is essential for compliance.
- Improving Model Performance: Understanding the decision-making process allows developers to identify and rectify flaws in AI models, leading to improved performance and fairness.
Several methodologies have been developed to enhance the interpretability of AI systems:
- Interpretable Models: Employing inherently interpretable models, such as decision trees and linear regression, provides transparency. These models are easier to understand but may not always capture complex relationships in data.
- Post-Hoc Explainability: Techniques like LIME (Local Interpretable Model-agnostic Explanations) and SHAP (SHapley Additive exPlanations) offer explanations for complex models after they have made decisions, aiding in understanding the contribution of each feature to the model’s predictions.
- Visualisation Tools: Visualising the decision-making process through heatmaps, feature importance plots, and decision trees can make AI outputs more understandable.
- Counterfactual Explanations: This approach explains model decisions by describing how changes in input features could lead to different outcomes, helping users understand the sensitivity of the model to various inputs.
The practical applications of XAI span multiple industries, enhancing decision-making and fostering trust:
- Healthcare: In diagnostic AI systems, XAI provides transparency, assisting doctors in understanding the reasoning behind AI-generated diagnoses and treatment recommendations. For instance, IBM Watson Health utilises XAI techniques to explain its cancer treatment recommendations to oncologists.
- Finance: Financial institutions leverage XAI to ensure transparency and fairness in credit scoring and loan approval processes. By offering clear explanations for credit decisions, banks can enhance customer trust and comply with regulatory requirements.
- Legal and Criminal Justice: XAI is employed to elucidate decisions made by predictive policing and judicial decision support systems, ensuring that AI systems do not perpetuate bias and that their decisions are legally and ethically sound.
Despite its advantages, implementing XAI presents certain challenges:
- Complexity vs. Interpretability:Achieving a balance between the complexity of AI models and their interpretability presents a formidable challenge. While advanced models such as deep neural networks can more capably represent intricate data patterns, they often lack clarity in their explanations.
- Standardisation: The lack of standardised methods for achieving explainability can lead to inconsistencies in how explanations are generated and interpreted.
- Performance Trade-offs: Simplifying models to achieve explainability may result in reduced performance, necessitating a careful balance between accuracy and interpretability.
— As AI reshapes business and society, transparency and accountability are no longer optional. Adopting Explainable AI (XAI) is essential for building trust, ensuring compliance, and making ethical, impactful decisions. Organisations that prioritise XAI will unlock AI’s potential while meeting rising expectations for fairness and clarity.