The 2024 Physics Nobel Prize: A Win for the Intersectorals
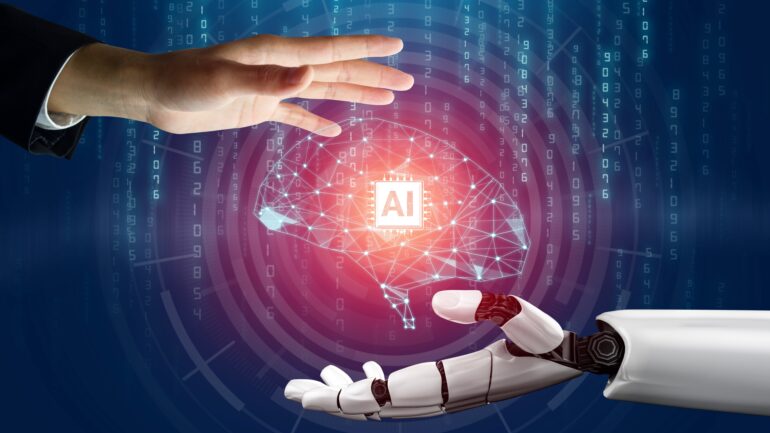
This year’s Nobel Prize in Physics was awarded to John Hopfield and Geoffrey Hinton, a pair of luminaries in both physics and artificial intelligence, for their groundbreaking contributions to our understanding of learning and information processing in neural systems. Their research bridged the gap between theoretical physics and neuroscience, paving the way for advances in AI that shape industries and economies today.
The 2024 Nobel Prize in Physics for John Hopfield and Geoffrey Hinton is a significant moment in the ongoing integration of AI and physics. While debates about the boundaries of scientific disciplines continue, this award acknowledges the groundbreaking importance of their contributions to both fields. Their work has changed how we think about learning, both in biological systems and in machines, and its impact will only deepen as AI becomes even more integral to scientific progress.
Yet, while this recognition places the duo’s work at the forefront of scientific achievement, it also stirs debate within the physics and AI communities about the scope and limits of this intersection.
The Breakthrough
The award citation highlights two main breakthroughs: John Hopfield’s development of his 1982 concept of ‘Hopfield networks’ and Geoffrey Hinton’s introduction of ‘Boltzmann machines’. These advances fundamentally changed our understanding of how information is processed in systems with many interacting elements, such as neurons in the brain.
Hopfield’s network models explained how memory could emerge from networks of simple, binary neurons. He demonstrated that the brain might be using physics-like principles to perform associative memory tasks, suggesting that each neuron contributes to the system’s collective behaviour, much like particles in thermodynamics.
Hinton extended these ideas by applying the probabilistic principles of the Boltzmann distribution to neural networks. His Boltzmann machine represented an early step toward the modern deep learning revolution, where the machine could ‘learn’ through energy-based optimisation. The machine minimised the system’s energy through repeated adjustments, a process akin to how natural systems tend to seek states of lower energy.
By linking neural computation with physics, the duo laid the conceptual groundwork for many machine learning algorithms we rely on today, from image recognition systems to large language models. Their contributions form a cornerstone of how we understand both biological intelligence and artificial neural networks, propelling innovations across various scientific fields.
Why It Matters
The significance of Hopfield and Hinton’s work cannot be overstated. In the 21stcentury, AI has become a cornerstone of technological progress, from autonomous vehicles to healthcare diagnostics. Their theoretical foundations in neural networks have accelerated AI research by connecting abstract physics principles with the learning behaviour of complex systems.
The Nobel committee’s decision also highlights how the boundaries between disciplines are increasingly porous. This win underscores that the conceptual tools of physics, once confined to understanding matter and energy, can be harnessed to decode biological and artificial intelligence. The blending of neuroscience and AI with physics reshapes how we view learning systems, suggesting that physical laws might govern the brain’s activity in ways we are just beginning to grasp.
Moreover, the practical applications of their work are far-reaching. AI systems, inspired by their models, now touch nearly every industry. Hinton’s recent work on deep learning helped unlock vast potential in natural language processing, robotics, and drug discovery. As AI continues to grow exponentially, the influence of these two pioneers will only become more pronounced in shaping future innovations.
The Critiques: Physics or AI?
However, the award has sparked critique from some quarters of the scientific community. A major point of contention is the Nobel Prize’s intent: to honour those who advance ‘physics’. Some argue that while Hopfield and Hinton’s contributions are undeniably transformative, they may lie outside the traditional bounds of physics. Is the Nobel committee, critics ask, overextending the field’s domain by awarding physics prizes for work that could equally fit under neuroscience or computer science?
This echoes past debates about other interdisciplinary winners. Hopfield and Hinton’s work is more closely associated with theoretical neuroscience and artificial intelligence than with the study of fundamental forces or particles. While Hopfield’s application of statistical physics to neural computation is well-grounded in physics, Hinton’s contributions, especially in deep learning, are often considered part of AI and computer science.
This critique raises questions about the evolution of scientific disciplines. As the frontiers of knowledge become increasingly interdisciplinary, where do we draw the line between physics and other domains? While some purists argue that the Nobel should focus on more traditional subjects like quantum mechanics or astrophysics, others celebrate the decision as a recognition of how physics principles apply to complex systems far beyond classical boundaries.
Why It Matters
Despite these critiques, this Nobel Prize is a reflection of the times. Interdisciplinary research, where the tools and insights from one field are applied to challenges in another, is becoming more and more vital to solving today’s biggest scientific and technological problems.
The award sends a message to the scientific community that ideas should not be confined to rigid silos. The fusion of physics, AI, and neuroscience has already yielded incredible advances in deep learning, and further collaboration could lead to even more groundbreaking discoveries. In the future, we may find that the principles Hopfield and Hinton discovered will apply not just to machine learning, but also to more fundamental questions about how our brains – and perhaps the universe itself – learn and adapt.
Additionally, with AI’s growing role in our daily lives, understanding its underlying principles is increasingly important. As systems become more autonomous, discerning the mathematical and physical structures governing their learning processes will be key to making these systems safer, more transparent, and more reliable.
Moving forward, this award may inspire further research that uses the principles of physics to explore biological systems, or even vice versa. We are in an era where disciplines that once seemed distinct now intersect, opening new possibilities for future innovation. Researchers will likely continue building on Hopfield and Hinton’s ideas, pushing the boundaries of AI even further by applying more sophisticated physical models to learning systems.
In awarding these pioneers, the Nobel committee signals the future of physics – and perhaps science itself – lies in interdisciplinary collaboration. The line between physics, neuroscience, and AI is becoming ever more blurred, and in that blur lies the potential for new discoveries that could reshape our world.