Harnessing AI for Smarter Portfolio Design
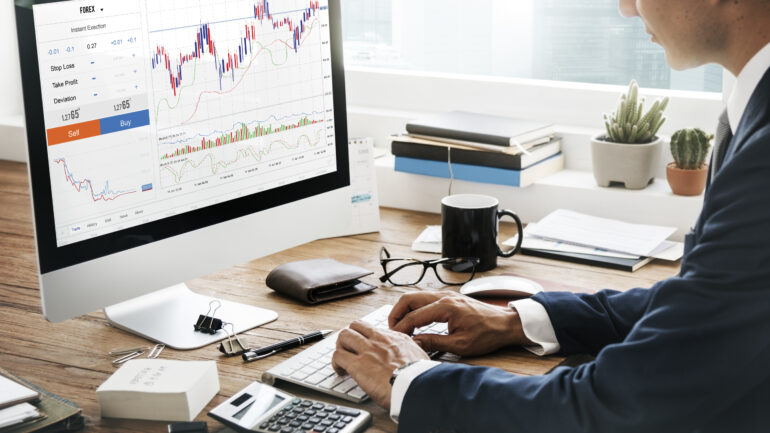
Large Language Models (LLMs) are revolutionising portfolio design by analysing financial markets with greater depth and speed than traditional methods. By synthesising vast datasets, recognising patterns, and making nuanced predictions, LLMs enable investors to better navigate market volatility and build more resilient, diversified portfolios.
The financial world has long relied on a mix of quantitative models and human expertise to design investment portfolios and predict market trends. However, the rise of Artificial Intelligence (AI), particularly Large Language Models (LLMs), is transforming how investors approach portfolio construction. These models, capable of processing vast amounts of unstructured data from news articles, earnings reports, and market trends, offer a level of market insight that was previously unattainable.
As financial markets become more complex, the ability to process and interpret large datasets in real time is a crucial competitive advantage. Investors can now rely on AI-powered models to not only forecast market shifts but also suggest portfolio adjustments in response to emerging risks and opportunities.
How LLMs Analyse Financial Markets
Traditionally, market analysis has focused on structured data – historical prices, earnings, interest rates, etc. LLMs, however, excel in analysing unstructured data like news reports, social media sentiment, and even geopolitical events, which are often leading indicators of market shifts. According to recent research from the University of Chicago, LLMs can process and synthesise diverse information sources, providing insights into underlying market dynamics that human analysts may overlook.
By identifying subtle relationships between market events and asset performance, LLMs can generate predictive signals that guide investment decisions. For instance, an LLM may recognise that specific economic policies in emerging markets correlate with commodity price movements, enabling portfolio managers to adjust allocations accordingly.
One of the most compelling impacts of LLMs is their ability to design proactive investment portfolios. Traditional models often react to past performance data, but LLMs can anticipate future trends by analysing market sentiment and historical patterns. This predictive capability helps investors mitigate risks more effectively and take advantage of opportunities before they are widely recognised by the broader market.
For example, LLMs can evaluate how investor sentiment around a company or sector evolves following quarterly earnings reports or geopolitical announcements. This allows them to make real-time suggestions to adjust portfolios, enhancing returns while minimising risks.
LLMs offer several key advantages when applied to portfolio design:
- Broader Data Integration: LLMs can ingest data from various sources, including news outlets, earnings call transcripts, and financial statements, providing a more comprehensive view of the market.
- Improved Predictive Accuracy: By understanding natural language, LLMs can interpret market sentiment and predict how it might influence asset performance in the near future.
- Real-Time Decision-Making: AI models can update portfolio recommendations in response to breaking news or emerging risks, allowing investors to act swiftly in volatile markets.
- Minimised Human Bias: While human analysts can be influenced by cognitive biases or emotions, LLMs offer objective, data-driven insights that can lead to more balanced portfolios.
- Scenario Analysis: LLMs can simulate different market conditions, helping portfolio managers understand how various strategies might perform under different economic environments, such as recessions or booms.
The Future of Investment Management
While LLMs have demonstrated great promise in financial market analysis, they are not without limitations. AI models can sometimes struggle with the inherent unpredictability of financial markets, particularly during extreme events like market crashes or black swan events, where historical data and sentiment analysis may provide limited guidance.
Furthermore, LLMs are not immune to errors, particularly when interpreting ambiguous or biased data. As Kiplinger notes, the reliance on AI should be balanced with human oversight, as the context and nuances of certain market events may require human intuition and expertise.
As AI grows, its role in portfolio design and financial analysis will likely expand. LLMs will increasingly work alongside human portfolio managers, augmenting their decision-making processes by providing deeper market insights. The ability to quickly synthesise vast datasets, uncover hidden patterns, and react to real-time developments will make AI-driven portfolio management indispensable to the modern investor.
Financial institutions are already exploring the use of AI in constructing robo-advisors, which can offer personalised investment advice based on market trends, risk tolerance, and long-term financial goals. Over time, the integration of LLMs into mainstream financial tools could democratise access to advanced market analysis, empowering individual investors with capabilities that were once limited to large institutional firms.