Is a Model Collapse Imminent
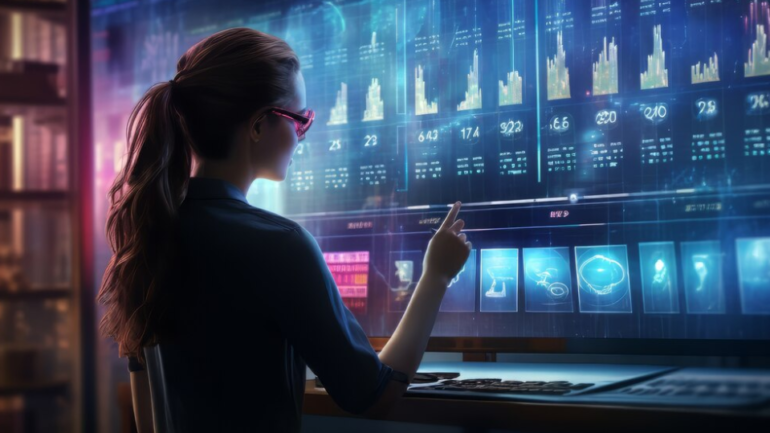
A recent report by Stanford University points out how the almost insatiable hunger for data by LLMs has raised a rather alarming possibility of the Internet running out of data to train these models
A significant proportion of recent algorithmic progress, including progress behind powerful LLMs (Large Language Models), has been achieved by training models on increasingly larger amounts of data, according to the latest research AI Index Report published by Stanford University. ChatGPT 3.5 model was trained using text databases from the Internet. This encompassed a massive 570GB of data sourced from published books, webpages, Wikipedia entries, random online articles as well as other public domain textavailable on the Internet. Statistics reveal that a total of 300 billion words were fed into the system.
The almost insatiable hunger for data by LLMs has raised a rather alarming question about the Internet running out of data to train these models. The Stanford AI Index report confirms a recent observation by Jack Clark, an AI Index Steering Committee member andco-founder of Anthropic – the San Francisco-based AI safety and research company, that foundation models have been trained on meaningful percentages of all the data that has ever existed on the Internet.
Low-quality language data might run out by 2030
The increasing reliance on data by AI models has raised concerns about potential data scarcity for future computer scientists – preventing further enhancementsto future systems. Epoch, an AI research firm, predicts that within 2030 and 2050, the supply of low-quality language data will be exhausted, followed by high-quality language data before 2026, and vision data by 2030 to 2060. This might slow down ML progress. “All of our conclusions rely on the unrealistic assumptions that current trends in ML data usage and production will continue and that there will be no major innovations in data efficiency. Relaxing these and other assumptions would be promising future work,” Epochclarified.
High-quality language stock might run out this year
Researchers at Epoch have conducted projections, both historical and compute-based, to determine when AI researchers might exhaust all available data for training. The historical projections rely on observed growth rates in the sizes of data utilised for training foundational models. The compute projections, on the other hand, adjust the historical growth rate by considering projections of available compute resources. Thus, the researchers estimate that the supply of high-quality language data could be exhausted by 2024, low-quality language data within the next two decades, and all image data by the late 2030s to mid-2040s.
In theory, the issue of limited data availability can be tackled by utilising synthetic data, which refers to data generated by AI models themselves. For instance, one LLM can be trained using text generated by another LLM. The use of synthetic data for training AI systems holds much promise as a means to address potential data scarcity.It also offers the possibility to generate data in scenarios where naturally occurring data is scarce, such as for underrepresented populations.
Limitations of Synthetic Data
In the past, there was limited understanding on the feasibility and efficacy of utilising synthetic data for training generative AI systems. However, recent research points out the limitations associated with training models using synthetic data. For example, a group of researchers from the UK and Canada found that models primarily trained on synthetic data are prone to collapse. This involves the gradual loss of the models’ ability to capture the true underlying data distributions, leading to a restricted range of outputs.
With each subsequent generation trained on additional synthetic data, the model produces an increasingly limited set of outputs. With an increase in the number of synthetic generations, the extremities of the distributions diminish, and the density of generations shifts towards the mean. This trend implies that over time, models predominantly trained on synthetic data produce less diverse and less widely distributed results.This research underscores the continued importance of human-generated data for training capable LLMs that can produce a diverse array of content.
Need for Data Management Systems
To address these challenges, companies must find ways to access and utilise large amounts of data. One approach is to invest in data management systems and develop data governance policies that enable efficient handling of data. Additionally, companies can adopt data minimisation strategies, which focus on collecting only the necessary data and deleting it once it is no longer needed.
Data privacy is another critical issue that must be considered. As AI systems become more integrated into our daily lives, organisations must ensure they can explain the decision-making process behind their AI tools. This involves understanding the factors influencing the algorithm’s outcomes and communicating them clearly to users. Organisations should invest in developing and implementing explainable AI models, provide training to employees, and foster a culture of transparency and accountability.
Acknowledgements: