The Promise and Pitfalls of Large Language Models in the Workplace
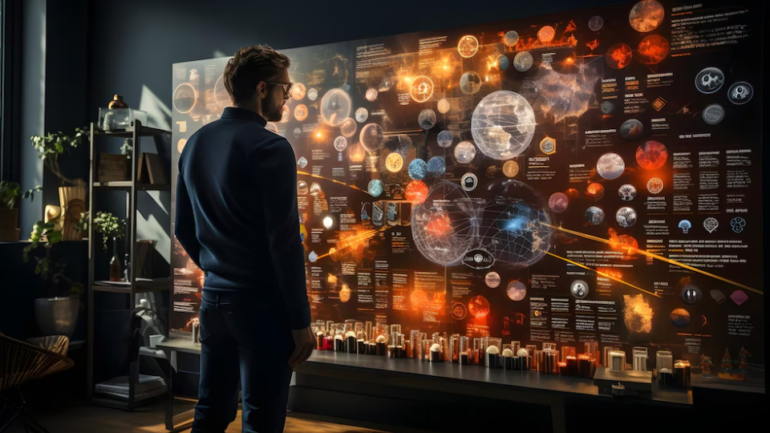
While costly and complex to develop, LLMs offer users the promise of performing language-based tasks with efficiency and scale previously limited to human capability. But are they truly the job-replacing titans some predict them to be?
Large Language Models (LLMs) today have emerged as a major technological breakthrough, propelling our abilities to generate text and images in response to diverse prompts to unprecedented heights. These sophisticated tools, while costly and complex to develop, offer users the promise of performing language-based tasks with efficiency and scale previously limited to human capability. But are they truly the job-replacing titans some predict them to be? As organisations integrate these AI behemoths into their workflows, they encounter a stark truth: human intuition and oversight remain indispensable.
LLMs at Work: Beyond the Hype
While LLMs are already transforming certain tasks with their capacity to process and interpret data, their adoption within companies is still in its infancy, marked by cautious exploration and a recognition of the intricate balance between artificial intelligence and human expertise.
Yet they are not merely theoretical marvels; they’re already in action in industries, digesting vast amounts of data to summarise content, craft reports, and extract vital information. They’re the artists behind the curtain, creating images with tools like Dall-E 2, and the silent partners generating synthetic data for voice recognition systems such as Amazon’s Alexa. Yet, their role in the corporate world is still nascent, largely experimental, and riddled with challenges.
Consider the case of Audi. The automobile giant has piloted a customised LLM-powered chatbot, designed to sift through databases in real time, providing employees with critical information. It’s a bold step into the AI arena, but one that requires careful navigation through the complexities of LLMs’ ‘black box’ nature.
The Major Challenges of Integrating LLMs
Organisations eager to harness LLMs face a series of hurdles that underscore the continued need for human input:
- Knowledge Capture: The ability of LLMs to process proprietary information and generate insights hinges on the quality of data fed into them. Sifting through organisational knowledge to find valuable data is a monumental task, often requiring new roles like data librarians.
- Output Verification: The outputs of LLMs, particularly in non-programming tasks, demand human judgment to determine their adequacy. Without proper scrutiny, the risk of unverified information shaping decisions is high.
- Output Adjudication: LLMs may produce varying results from the same data set, leading to internal conflicts. Organisations must navigate these discrepancies, deciding which outputs align best with their goals.
- Cost-Benefit Analysis: The actual benefits of LLM implementation can be unpredictable and context-dependent. Savings in one area may be offset by new costs or the need for additional oversight in another.
- Job Transformation: The integration of LLMs into the workforce is unlikely to result in sweeping job cuts. Instead, it may lead to role evolution, where employees adapt to new responsibilities alongside their AI colleagues.
Forging Ahead with LLMs
For managers, the path forward involves setting standards for LLM use, centralising AI operations to manage quality and consistency, and educating employees on the capabilities and limitations of these tools.
The road to AI integration is long and winding, with each organisation finding its pace. As we venture further into this technological terrain, the need for a human touch remains a constant companion. The true potential of LLMs will be realised not in replacing the human workforce but in augmenting it, fostering a collaborative future where machine intelligence and human ingenuity coalesce to drive innovation and productivity.
As we continue to grapple with the implications of LLMs in the workplace, it is clear that they will not render humans obsolete. Instead, they will redefine the nature of work, demanding a recalibration of skills and a reimagining of the human-AI partnership. The journey is just beginning, and its trajectory will be charted by those who recognise the value of both artificial intelligence and the irreplaceable human spirit.
Based on a paper published in the MIT Sloan Management Review, dated 4 March 2024.
Know more about the syllabus and placement record of our Top Ranked Data Science Course in Kolkata, Data Science course in Bangalore, Data Science course in Hyderabad, and Data Science course in Chennai.